MM021 – Unconventional Uses of Data Science
Main business applications of Advanced Analytics and Data Science are pretty much known thanks to the large volume of publications and case studies, but what can we tell about non-business ones? Here are three “uncommon” applications of Advanced Analytics and Data Science one may not think they are used for.
Natural Event Prediction
Floods, earthquakes, hurricanes, volcanic eruptions and other natural disasters are pretty much tough to model and even more difficult to predict. Therefore, in order to minimize the loss of human life and the property damage associated with these massive events, Machine Learning methods have been recently used to contribute in the advancement of prediction systems, with the aim of providing more accurate and cost-effective solutions.
To feed predictive algorithms, however, another major problem to be solved is data availability. Because of high operating costs in fact, data is generally insufficient to foresee such events.
Previous events are a primary source of information to help Machine Learning algorithms to understand the key factors that mostly contribute to or which events occur before a natural disaster. Once these events and features are identified, they are used to train other algorithms with the aim of increasing the prediction accuracy.
In order to overcome data scarcity, experts are trying to combine data collected from conventional sources like satellites, drones, weather stations and equipment (e.g. marine sensors, seismographs) with data coming from unconventional ones. Those latter sources include Social Sensor Data (i.e. data provided by people posting on social media in case of shocking events), as well as data collected by studying the behavior and movement of animals, which as it is acknowledged, are able to better feel the vibrations of the earth.
Vaccines Development
It may look some sort of biohazardous matter but actually, Advanced Analytics might provide a tremendous value to fight viral diseases like flu. Contrary to popular belief in fact, it is not bacteria, but rather viruses which mainly cause illnesses. Figures tell us that while just 1% of the former is dangerous for our health, around 99% of the latter is harmful.
How does a so small being manage to cause potentially heavy damage? Well, they basically infiltrate in our cells and they start replicating over and over causing the disease to break out.
To fight against those menaces, vaccines have been tuned to allow our organism to develop specific antibodies and eliminate the disease.
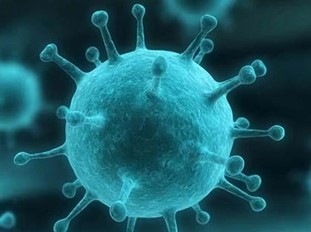
Now, let’s consider the case of flu. As viruses change continuously and evolve over time, it is difficult to find a vaccine to combat all possible circulating types of the disease. Thus, one or more strains of the virus have to be chosen when formulating the vaccine and its effectiveness will closely depend on which virus will be dominant during the next flu season. If the selected strains will somehow resemble the circulating viruses the effectiveness will be high.
This is where data Science and Analytics come into play. Predicting the future evolution of viruses is the current goal of scientists, as it would make the task of selecting the right vaccine much easier. Some algorithms used to perform phylogenetic analyses (i.e. understand the evolution of a genetically related group of organisms studying their protein or gene evolution) and to try to anticipate viruses’ behavior are non-negative least squares, maximum likelihood trees or scoring methods.
In the future, should this approach result effective, it could be extended also other fields, like cancer cell evolution and to combat pathogen resistance to drugs.
Sport Analytics
Even sport can’t escape the impact of Advanced Analytics. As sporting organization can leverage huge amounts of data, Machine Learning is used in order to improve decision both on and off the field, from selling more tickets, to increase athlete’s performance, as well as to reduce players injuries and guess which youth player has the potential to be the star Messi or Ronaldo.
As far as on the field activities are concerned, an example is surely Liverpool FC, whose research division takes this approach to a great extent through a concept labelled pitch control. By leveraging on data, they are able to split the pitch in areas where players are much more likely to get to before the opponent team, and therefore Liverpool players are advised to pass the ball in those areas, rather than in those controlled by the rival players. In such way, they can also estimate the probability of scoring depending on the action.
NBA as well is heavily leveraging on data analytics with full-time staff departments in order to support coaches and tacticians in their training. The Golden State Warriors for example, which are among the dominant teams in the last seasons, has one of the NBA’s most effective analytics unit.
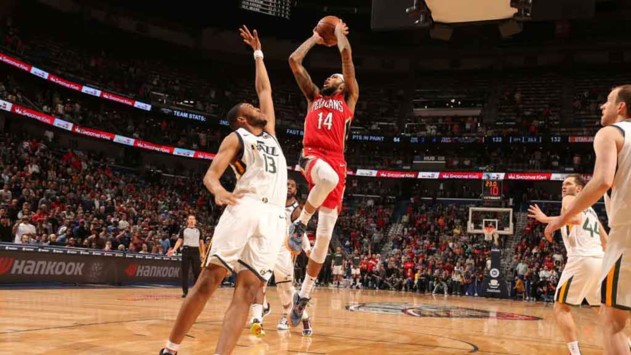
However, the massive resort to Data Analytics is triggering some aversion by fans, as data analysts are accused of disregarding the emotional level of the game, risking giving up spontaneity and fun, limiting players’ freedom of self-expression on the field.
The examples outlined above are just a tiny portion of the entire universe of application of Advanced Analytics and Data Science. The potential for other applications is in fact huge. Currently, they range for instance from Energy Exploration, to Neuroscience, or from Computational Physics to Space Weather prediction.
References:
Mosavi, Amir & Ozturk, Pinar. (2018). Flood Prediction Using Machine Learning Models: Literature Review. Water. 10. 1536. 10.3390/w10111536.
https://365datascience.com/influenza-vaccines-data-science/
https://www.sciencedirect.com/topics/medicine-and-dentistry/phylogeny
https://hackernoon.com/9-unusual-problems-that-can-be-solved-using-data-science-e7dbb89aa0c4
https://biztechmagazine.com/article/2018/12/how-data-analytics-revolutionizing-sports
https://www.theguardian.com/sport/2018/aug/16/sports-nerds-analytics-data
https://www.bnova.it/blog/big-data-advanced-analytics/
http://deeptechwire.com/using-big-data-to-predict-natural-disasters/
(banner image taken from “The Day after Tomorrow” movie)